AI Tools Detect Parkinson’s
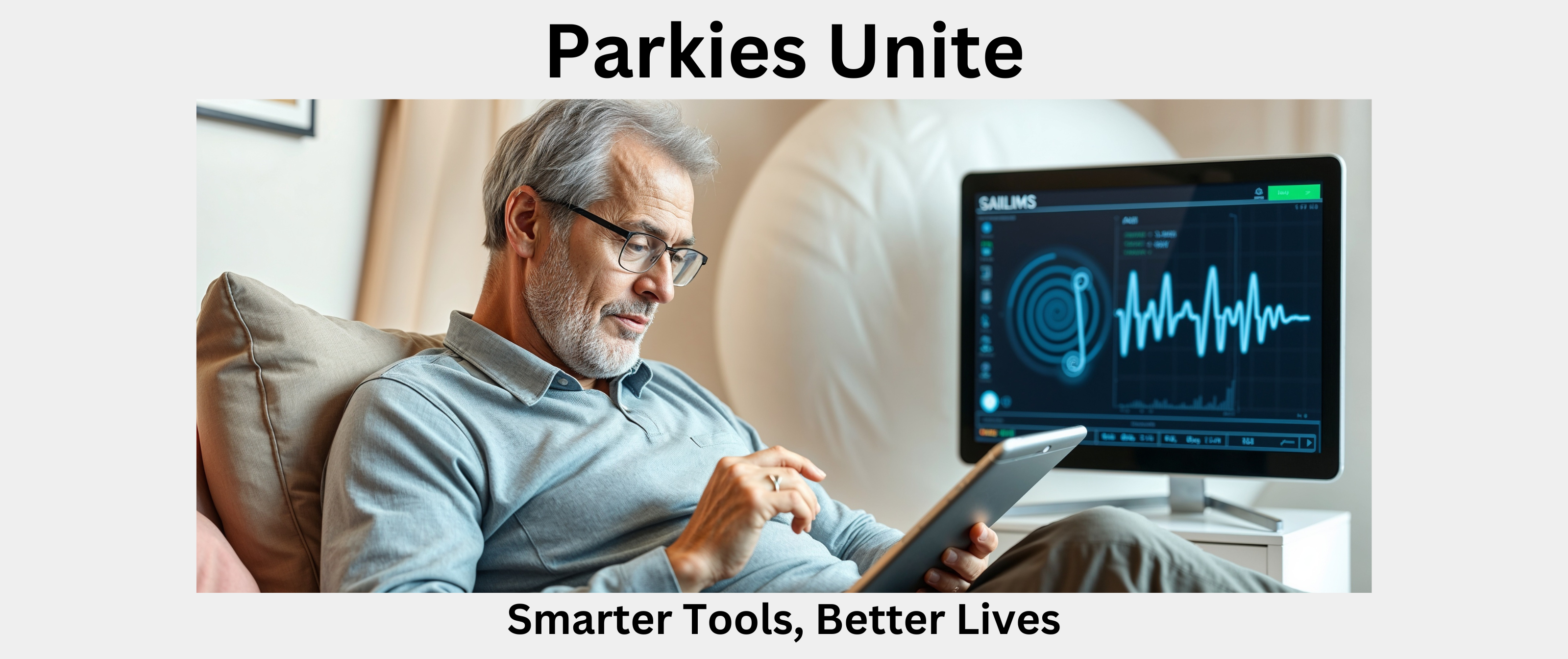
Parkinson’s disease (PD) is a growing global health challenge, affecting around 10 million people worldwide and projected to impact 20 million by 2050. As a progressive neurological condition marked by both motor (tremors, rigidity, bradykinesia) and non-motor symptoms (anxiety, sleep issues, cognitive decline), early diagnosis is critical—but remains difficult due to variability and subtlety in symptom presentation. Fortunately, recent advances in Artificial Intelligence (AI), specifically Machine Learning (ML) and Deep Learning (DL), are transforming how Parkinson’s is detected and monitored.
In this post for Parkies Unite, we dive deep into a 2025 review titled “A Review of Machine Learning and Deep Learning for Parkinson’s Disease Detection”, unpacking how AI is revolutionizing diagnosis, what datasets and models are being used, and what challenges must still be tackled for real-world impact.
Keywords for Context:
Parkinson’s disease, early detection, machine learning, deep learning, gait analysis, digital biomarkers, wearable sensors, speech analysis, MRI analysis, convolutional neural networks, UPDRS scoring, artificial intelligence, PD diagnosis, federated learning, data preprocessing, neuroimaging, voice biomarkers, explainable AI, CNN models, data augmentation.
Why Early Parkinson’s Diagnosis Is Difficult
PD is a complex degenerative disease. Symptoms—ranging from tremors and rigid muscles to fatigue, constipation, and sleep disruption—can present differently in each person. Emotional changes such as depression and anxiety often appear early. While medications and treatments help, there’s no cure.
Traditional diagnosis relies heavily on clinical judgment and imaging, often resulting in late-stage detection. This delay reduces treatment efficacy and limits quality of life. Hence, there’s a massive drive toward AI-supported tools that can spot PD earlier and more accurately.
The Role of Machine and Deep Learning
What’s the Difference?
- Machine Learning (ML): Algorithms that can find patterns in data, such as support vector machines (SVM), decision trees, or XGBoost.
- Deep Learning (DL): A subset of ML using neural networks (like CNNs and RNNs) to model complex patterns, especially useful for images and time-series data.
These technologies offer predictive modeling based on speech, handwriting, gait, neuroimaging, and even mobile sensors. When trained on large, quality datasets, they outperform human judgment and traditional diagnostics—sometimes reaching accuracies above 99%.
Publicly Available Parkinson’s Datasets
Here are some key datasets that fuel these AI systems:
- UCI Parkinsons Dataset: 197 voice recordings, 23 with PD.
- Italian Parkinson’s Voice & Speech: Audio samples across different age groups and vocal tasks.
- Cerrahpasa Voice Dataset: Includes UPDRS scores for regression modeling.
- Parkinsons Telemonitoring: Over 5,800 home voice recordings to predict symptom progression.
- PPMI (Parkinson’s Progression Markers Initiative): A comprehensive open-access clinical and biomarker dataset.
- AMP-PD: Protein expression and mass spectrometry data.
- mPower: Mobile data from 960 participants measuring gait, tapping speed, memory.
- NewHandPD & HandPD: Handwriting samples captured with smart pens and tablets.
- MDVR-KCL: Voice samples labeled with Hoehn & Yahr and UPDRS scales.
- Gait in Parkinson’s Dataset: Force plate data for walking analysis using wearable sensors.
Each dataset brings its own modality—voice, handwriting, gait, imaging, or clinical scores—providing unique input for training AI models.
Data Preprocessing: Making Raw Data AI-Ready
Raw medical data is often messy—filled with noise, imbalances, and missing entries. Preprocessing improves model accuracy and reliability.
Common Techniques Include:
- Standardization & Normalization: Aligning data scales.
- Data Augmentation: Using GANs, spectrograms, or transformations to expand datasets.
- Feature Engineering: Extracting relevant signals (e.g., MFCC from audio).
- Dimensionality Reduction: Via PCA to reduce noise and improve learning.
- Balancing Techniques: Using SMOTE or oversampling to address class imbalance.
- Online Learning: Using Incremental PCA and DBN-ANFIS for evolving predictions.
These methods ensure ML and DL models learn meaningful patterns rather than noise or outliers.
Machine Learning Approaches in Action
Many studies used supervised learning with stunning success:
- XGBoost: Achieved up to 98.75% accuracy on voice biomarkers.
- Random Forest (RF): Performed with 92% accuracy on handwriting spiral tasks.
- K-Nearest Neighbors (KNN) and Naive Bayes: Effective in classifying acoustic features.
- Stacking Ensemble Models: Combining models like SVM + RF + logistic regression for even better predictions.
- Support Vector Machines (SVM-MFW): Classifies PD with 98.3% accuracy using DaTSCAN and biomarker data.
Some methods even predicted quality-of-life deterioration a year in advance using only UPDRS scores at three earlier timepoints.
Deep Learning Techniques: Going Deeper
DL thrives on multimodal data. These architectures lead the way:
- CNN-LSTM hybrids: Process speech spectrograms, identifying early vocal biomarkers.
- EfficientNet-V2: Analyzes MRI scans with 99.23% accuracy.
- ZF-Net with Optimization Algorithms: Classifies PD using voice features with up to 94.5% accuracy.
- GhostNet + ResNet-152: Processes EEG signals for 99% F-score.
- Transfer Learning with VGG19: Classifies handwriting and voice for perfect accuracy in some settings.
These models leverage everything from handwriting patterns to MRIs to voice recordings—offering non-invasive, accessible tools for patients and researchers.
Integration with Wearables and At-Home Tools
The future of PD detection lies in real-time, continuous data collection via wearables and smartphones. Gait data collected from foot sensors, tapping speed via touchscreen, and phonation analysis via microphones allow for personalized, frequent assessments from the comfort of one’s home.
Studies like mPower, NewHandPD, and Telemonitoring trials prove this is feasible. Wearables provide granular insights into moment-to-moment symptom changes, ideal for tracking fluctuations between “on” and “off” states.
Limitations and Future Directions
Despite incredible progress, challenges remain:
- Data Privacy: Health data is sensitive; anonymization and secure platforms like Federated Learning are needed.
- Data Scarcity: Large, multimodal datasets are rare. Cross-modality (voice + handwriting for same patient) is almost non-existent.
- Bias and Generalizability: Data often skewed by geography, gender, or age group.
- Black Box Models: Many DL models lack interpretability. Explainable AI (XAI) is essential for clinical adoption.
With the rise of wearable tech, cloud platforms, and privacy-focused machine learning, these hurdles are being addressed—but slowly.
Final Thoughts
This 2025 review makes it clear: AI is not just a future possibility—it is reshaping how we detect and manage Parkinson’s today. From smart pens to mobile phones and MRIs to voice apps, ML and DL offer clinicians new tools for faster, more accurate, and potentially earlier diagnosis.
For people living with PD, this means greater empowerment, personalized care, and better outcomes. For researchers, it’s a call to action to build better datasets, design more transparent models, and collaborate across disciplines.
Let’s embrace the potential of AI—responsibly, ethically, and inclusively.
Photo-realistic AI Image Prompt:
A middle-aged man sitting at a home workspace using a tablet with a spiral drawing test on screen, while a wearable gait sensor is strapped to his ankle. A digital assistant interface showing voice waveform is visible on a second screen nearby. Bright natural lighting, modern yet lived-in home environment, subtle medical cues like pill organizers or walking cane in background.
Taglines:
- Detecting PD with AI
- Parkinson’s Meets Technology
- Smarter Tools, Better Lives
Negative Prompt:
Malformed limbs, extra limbs, mutated hands, disfigured face, bad anatomy, malformed hands, Text, lettering, captions, generating images with text overlays
AI-generated medical infographics on Parkinson’s symptoms, treatment advances, and research findings; I hope you found this blog post informative and interesting. www.parkiesunite.com by Parkie
Keywords: AI diagnosis, Parkinson’s tools, digital biomarkers, speech detection, wearable monitoring