AI Wearable Sensors for Parkinson’s Disease
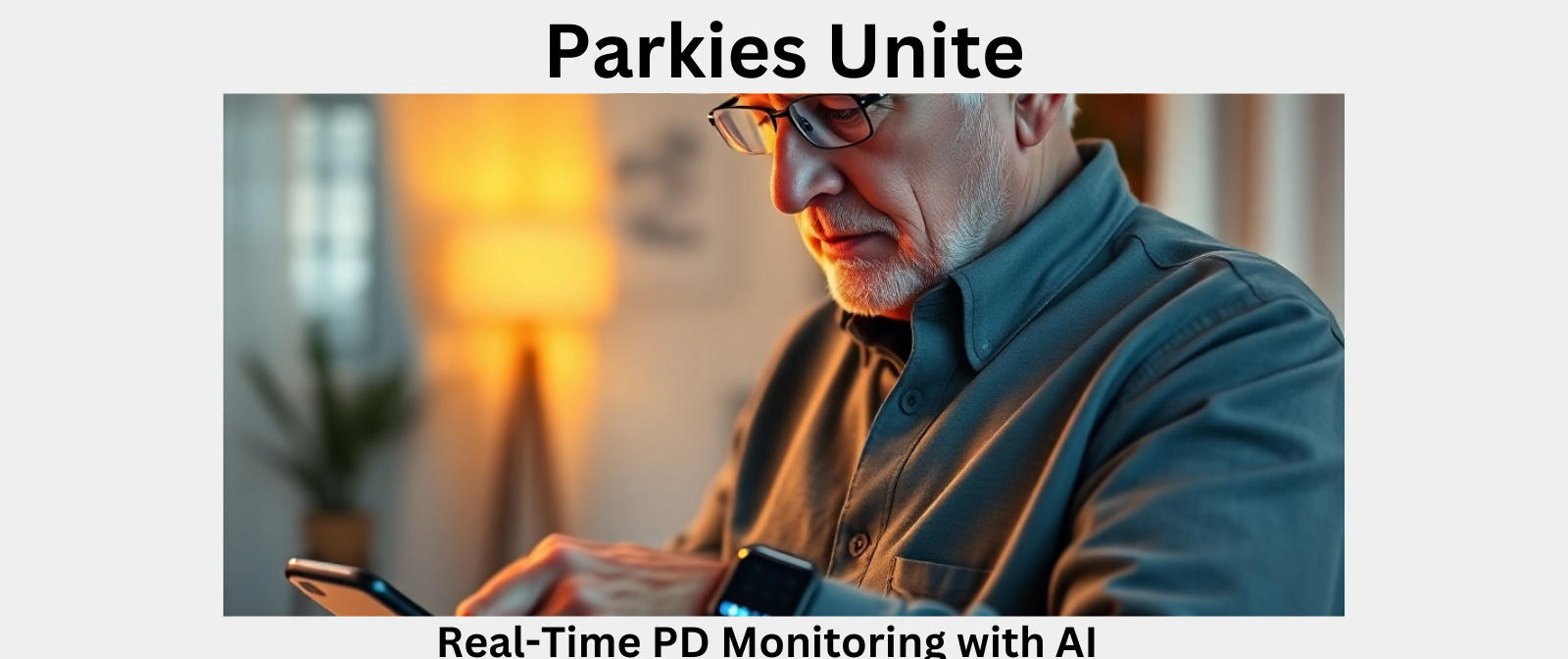
Introduction
Parkinson’s disease (PD) is a complex neurodegenerative disorder affecting millions worldwide. Traditionally, PD diagnosis and symptom tracking have relied on clinical observation tools such as the Unified Parkinson’s Disease Rating Scale (UPDRS) and Hoehn & Yahr (H&Y) scale. However, these methods have significant limitations, particularly in detecting “On-Off” fluctuations caused by dopamine-based treatments. With advancements in wearable sensors and AI-driven models, Parkinson’s disease management is undergoing a transformation, offering real-time, objective, and personalized tracking of symptoms.
The Limitations of Traditional Parkinson’s Assessment
1. Overreliance on Subjective Clinical Scoring
UPDRS Challenges:
- Subjectivity & Inter-Rater Variability – Different neurologists may score the same symptoms differently.
- Lack of Real-Time Tracking – Symptoms fluctuate throughout the day, but assessments occur only every few months.
- Limited Sensitivity for Early Diagnosis – Subtle pre-motor symptoms (sleep disturbances, gait changes, cognitive changes) may not be reflected in early UPDRS scores.
- Medication Influence on Scoring – Levodopa can temporarily mask symptoms, leading to inaccurate assessments.
H&Y Scale Challenges:
- Oversimplification of Symptoms – Classifies patients into broad stages without considering symptom nuances.
- Limited Predictive Value – Does not account for non-motor symptoms such as mood changes and cognitive decline.
- Poor Reflection of Motor Fluctuations – Does not assess medication effects, dyskinesia, or freezing episodes.
Understanding “On-Off” Fluctuations in Parkinson’s Disease
Parkinson’s patients often experience unpredictable variations in motor function due to dopaminergic medication effects. These fluctuations take different forms:
1. Wearing-Off Phenomenon
- Gradual return of symptoms before the next medication dose.
- Caused by the brain’s declining ability to store and release dopamine efficiently.
2. Sudden and Unpredictable Fluctuations
- Rapid switching between mobility (“On” state) and rigidity/tremor (“Off” state), often unrelated to medication timing.
3. Dyskinesia (Involuntary Movements)
- Excessive movement due to overstimulation of dopamine receptors.
- Common in long-term Levodopa users.
These fluctuations significantly impact daily life, yet traditional assessment tools fail to capture them effectively. AI-powered wearable sensors address these challenges by offering continuous, real-time monitoring.
How Wearable Sensors Are Transforming Parkinson’s Management
1. Types of Wearable Sensors
A. Accelerometers & Gyroscopes for Movement Tracking
- Detect tremor, bradykinesia (slowness), rigidity, and dyskinesia.
- Found in smartwatches, rings, and sensor patches.
- Example: Parkinson’s KinetiGraph (PKG) tracks bradykinesia every 2 minutes.
B. Smart Insoles & Gait Sensors
- Analyze stride length, freezing of gait (FOG), and balance issues.
- Example: Physilog 5 detects freezing episodes and fall risks.
C. Smart Gloves & Rings for Fine Motor Tracking
- Assess handwriting difficulties, finger dexterity, and micrographia.
- Example: GyroGlove stabilizes hand tremors.
2. AI-Powered “On-Off” State Detection
Wearable sensors use machine learning (ML) and deep learning (DL) algorithms to detect “On-Off” fluctuations. The system follows this process:
Stage | Process | Example Technologies |
---|---|---|
Data Collection | Sensors record movement (tremor, rigidity, gait) | PKG, smartwatches, smart insoles |
Preprocessing | AI removes noise & extracts movement features | Accelerometer & gyroscope data analysis |
Classification | AI predicts “On-Off” states | CNN, LSTM, SVM models |
Clinical Application | Neurologists adjust medication schedules | AI-based dosing recommendations |
Clinical Applications of Wearable AI in Parkinson’s Disease
1. AI-Powered Medication Adjustment
- AI predicts “Off” episodes before they happen, allowing timely medication adjustments.
- Random Forest models trained on PKG data predicted “Off” states 30 minutes in advance with 94% accuracy.
2. Fall Prevention & Smart Alerts
- Gait analysis wearables detect balance changes and send alerts before a fall occurs.
- LSTM-based models improved freezing of gait detection by 32%.
3. Digital Biomarkers for Clinical Trials
- AI-generated biomarkers enable objective symptom tracking, reducing reliance on subjective reports.
- Case Study: mPower App collected 3,700 movement records, detecting tremor 6 months earlier than clinical assessments.
The Future of AI-Powered Wearables in Parkinson’s Disease
1. Multimodal Sensor Integration
- Future wearables will combine gait, voice, tremor, and heart rate data for comprehensive PD assessment.
- AI models integrating smartwatch + gait analysis predict freezing of gait with >99% accuracy.
2. Closed-Loop Drug Delivery Systems
- AI-driven wearable pumps will automatically adjust dopamine therapy based on real-time symptom tracking.
3. Brain-Machine Interfaces (BMIs) & Neural Implants
- AI-enhanced brain stimulation devices could provide real-time PD symptom control.
Final Thoughts
Wearable AI sensors are transforming Parkinson’s disease management, offering real-time, objective, and predictive insights. As technology advances, closed-loop AI systems may eliminate motor fluctuations, enabling personalized treatment and improved quality of life.
AI-generated medical infographics on Parkinson’s symptoms, treatment advances, and research findings; I hope you found this blog post informative and interesting. www.parkiesunite.com by Parkie
Generative AI Prompt for an Image:
“A photorealistic image of an elderly man wearing a smartwatch and a ring sensor, sitting in a well-lit modern home, analyzing Parkinson’s disease data on a smartphone app. The wearable devices subtly glow with futuristic AI-enhanced tracking. The environment is warm and inviting, symbolizing technological innovation enhancing quality of life.”
Taglines:
- “AI Wearables: Redefining Parkinson’s Care”
- “Smart Sensors, Smarter Parkinson’s Tracking”
- “Real-Time PD Monitoring with AI”
Negative Prompt:
“Malformed limbs, extra limbs, mutated hands, disfigured face, bad anatomy, malformed hands, Text, lettering, captions, generating images with text overlays.”